Texas A&M Researcher Charts A New Direction In Biology, Quantum Computing
Story by Courtney Price, VMBS Marketing & Communications
Texas A&M researchers are using new computing technology to predict gene expression, which may be the key to targeted solutions for health issues like cancer and genetic diseases.

When most people think of relationships in biology, they probably think of big-picture, macro-level relationships such as the food chain or symbiotic species that we can observe in the world around us.
But there are important biological relationships at the micro level, too. Even within a single cell, there are networks that rival the complexity of cities.
Most of us will never have the opportunity to observe these networks because of their small size, but the situation is different for scientists like Dr. James Cai, a professor in the Texas A&M College of Veterinary Medicine & Biomedical Sciences’ (VMBS) Department of Veterinary Integrative Biosciences (VIBS).
Cai conducts research at a unique crossroads between single-cell biology and quantum computing, a multidisciplinary field using quantum mechanics to solve complex problems faster than would be possible on classical computers.
“We interpret data in order to find patterns between data points,” Cai explained. “Finding the rules for these patterns tells us how one factor can cause another variable to change.”
Already, his team’s findings — published last year in npj Quantum Information — prove that quantum computing has a huge potential to improve cellular and genomics research, greatly expanding the possibility of research methodologies and new treatments and diagnostics.
At the moment, Cai’s team is the only one he knows of in the entire world doing research with quantum computing and single-cell biology. His Ph.D. student Cristhian Roman Vicharra, a research assistant in the Texas A&M Department of Electrical & Computer Engineering, is a key member who spearheaded the study behind last year’s publication.
“It’s a very new field,” Cai said. “Most people working in quantum computing have a physics background. And people on the biology side don’t usually understand how quantum computing works. You really have to be able to understand both sides.”
Cai’s research specifically looks at the patterns and relationships between genes in cells, a system called the gene regulatory network (GRN).
“The GRN is like a map that tells us how genes affect each other,” Cai said. “For example, if one gene in a population of cells switches on or off, then it may change another gene. If that one is what we call a driver gene, it may change three genes, or five, or 20 down the line.”
While Cai studies very small phenomena, the impacts of his work are huge.
“If you can predict gene expression through the gene regulatory network and understand how those changes translate to the state of the cells, you might be able to control certain outcomes,” Cai said. “For example, you might be able to inhibit the growth of cancer cells or stop them from dividing.”
Mapping The World Of Individual Cells
Dr. James Cai
“If you can predict gene expression through the gene regulatory network and understand how those changes translate to the state of the cells, you might be able to control certain outcomes. For example, you might be able to inhibit the growth of cancer cells or stop them from dividing.”
Prior to 2016, scientists didn’t have the technology to capture gene expression data for individual cells in large scale; instead, they used whole tissues, or large clusters of cells. Unfortunately, having so many cells together made it difficult to study gene expression at the level needed to really understand how genes work together.
“Once single-cell data was available, we had enough data points to apply machine learning, which uses algorithms to process large amounts of data,” Cai said. “If you only have three points, you don’t have enough information for analysis. However, if you have one million data points, you can start to interpret them.”
But there was a technological problem with scientists’ ability to map GRNs.
“We studied the GRN by comparing genes together,” Cai said. “But the algorithms could only handle comparing two genes at a time in what’s called a pairwise relationship.”
Cai explained that only comparing genes in pairs could result in misleading conclusions, since genes may operate in more complex relationships. If gene A activates and so does gene B, it doesn’t always mean that gene A is responsible for gene B’s change. In fact, it could be gene C changing both genes A and B.
“We needed a system that could simulate multiple genes’ intercorrelation at the same time,” Cai said.
That’s where quantum computing comes in.
“With traditional computing, data is processed in bits, which only have two states — on and off, or 1 and 0,” Cai said. “But with quantum computing, you can have a state called the superposition that’s both on and off simultaneously. That gives us a new kind of bit: the quantum bit, or qubit.
“Because of superposition, I can simulate both the active and inactive states for a gene in the GRN, as well as this single gene’s impact on other genes,” he said.
Quantum computing can even account for the probabilities that genes will be either on or off.
“It’s called entanglement,” Cai said. “Entanglement means there are two or more qubits whose states are interconnected — what happens to one will affect the others. We can entangle them in such a way that when gene A is active, gene B is most likely active at the same time. We can even set the exact percentages, like 70% on and 30% off.”
Small Cells, Big Data
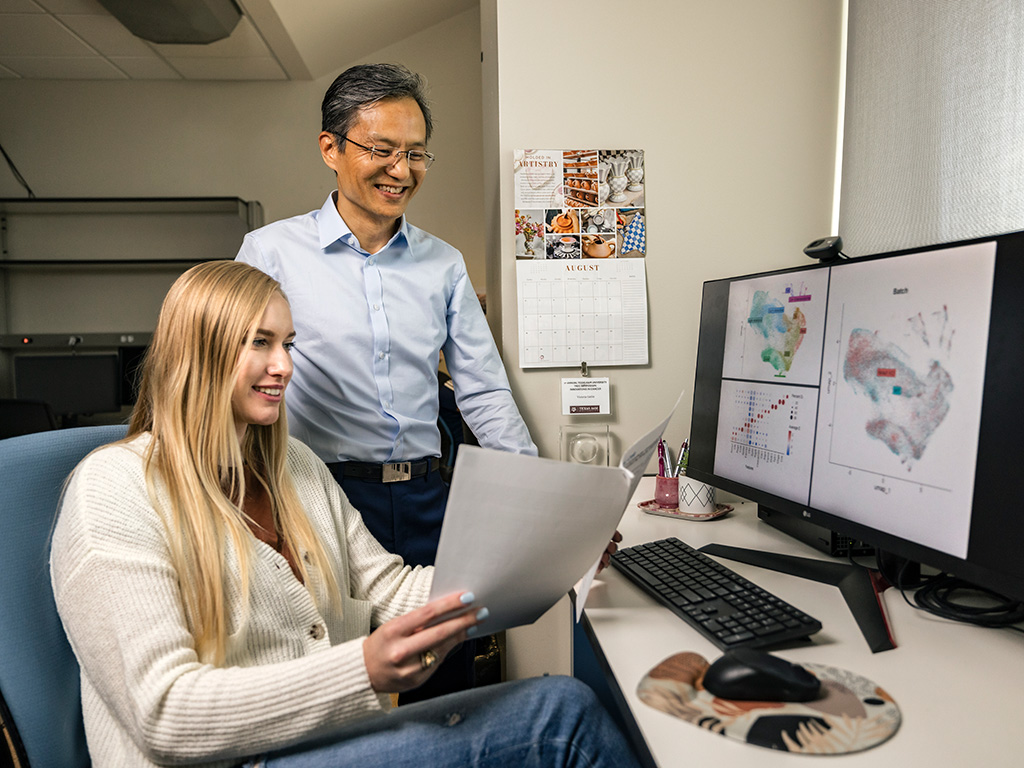
Once Cai and his team had the technology to map GRNs more accurately, they could begin to compare it with data from real cells.
“Let’s say you’re looking at cells of a certain type and you find a string of six genes that all activate at the same time,” Cai said. “We would represent that as 111111. But let’s say that in another cell of the same kind, those six genes are expressed differently — 011001. That means some are on and some are off.
“When you look for those six genes across millions of cells, you’ll see them in all kinds of combinations of on and off,” he said. “We can even have a frequency summary and say that 10% of the time they’ll be 111111 and 21% will be 011001. So now we can base our algorithms off data from these cells.”
Having accurate algorithms means Cai and his team can better predict the relationships between genes, making their GRN maps more accurate and, therefore, more useful for research. In fact, when the team published their data and findings in npj Quantum Information, it led to an unexpected discovery.
“Because our GRNs are constructed using different methods than traditional computing, we found some links between genes that people hadn’t known about previously,” Cai said. “Some researchers who specialize in the type of cells we studied read our paper and realized that our predictions using quantum computing fit their expectations better than the traditional model.”
While quantum analysis of GRNs is still in early development, the results are already quite promising.
“Hopefully we will find even more examples that give accurate predictions,” Cai said.
The Future Of Computational Single-Cell Biology
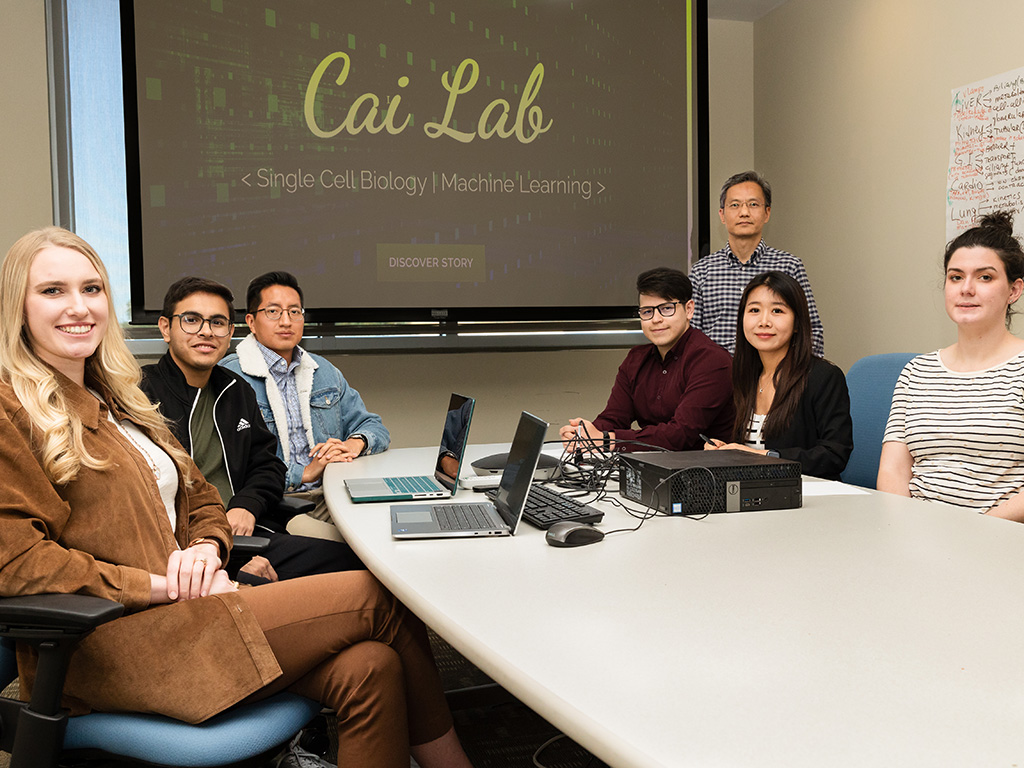
Right now, Cai and his team are focused on studying GRNs in healthy cells, but they’re not content to stop there.
“In the future, we plan to compare the healthy cells to ones with diseases or mutations,” Cai said. “We hope to see how a mutation might affect genes’ states, expression, frequencies, etc. That’s the next phase of the study.”
For now, it’s important to get as clear an understanding as possible of how healthy cells work before comparing them to non-normative cells.
“The first step is to predict this baseline model and see whether the network we’ve mapped makes sense,” Cai said.
As the field grows, Cai says there’s definitely a potential for translating computational biology research on single cells into a variety of applications, such as animal medicine.
“It all depends on the accumulation of knowledge,” he explained. “It takes time to map GRNs, but eventually, we hope that quantum computing may give researchers a quicker way to assess indirect relationships between genes, like the kinds of interactions that are less obvious. It could even be used to discover new uses for medications.”
One way that Cai is encouraging more people to engage with the field is through sponsoring the student organization QuantA&M, which was founded in 2022 to help undergraduate and graduate students learn more about the applications of quantum computing.
“We’re hoping to bring in external speakers and maybe teach a few workshops,” Cai said. “We’d really love for more students to get involved with quantum computing.”
###
Note: This story originally appeared in the Fall 2024 issue of VMBS Today.
For more information about the Texas A&M College of Veterinary Medicine & Biomedical Sciences, please visit our website at vetmed.tamu.edu or join us on Facebook, Instagram, and Twitter.
Contact Information: Jennifer Gauntt, Director of VMBS Communications, Texas A&M College of Veterinary Medicine & Biomedical Sciences, jgauntt@cvm.tamu.edu, 979-862-4216
You May Also Like